3.2.2.2 Delete Volume
The Delete Volume control allows you to remove a single volume from your DWI dataset. This is only recommended under the following circumstances: 1) You have severe artifacts in your data that post-processing cannot repair, such as motion within your DWI volume resulting in major signal dropouts, or an interleaved type of motion artifact, and 2) Your data has adequate over-sampling that removing volumes does not ill-define your tensor matrix (i.e. if you only acquire 6 directions without any replicates, you cannot remove any volumes). Under this circumstance, it may be advisable to remove DWIs containing artifacts. However, we recommend extreme caution in removing data, as the number of images in your dataset can affect the value of measures such as fractional anisotropy1,2. If you feel you must remove a large number of volumes for your data to be usable, consider carefully it's inclusion in statistical analyses.
The Delete Volume button is available in the Display Raw Image function of DIFF_CALC.
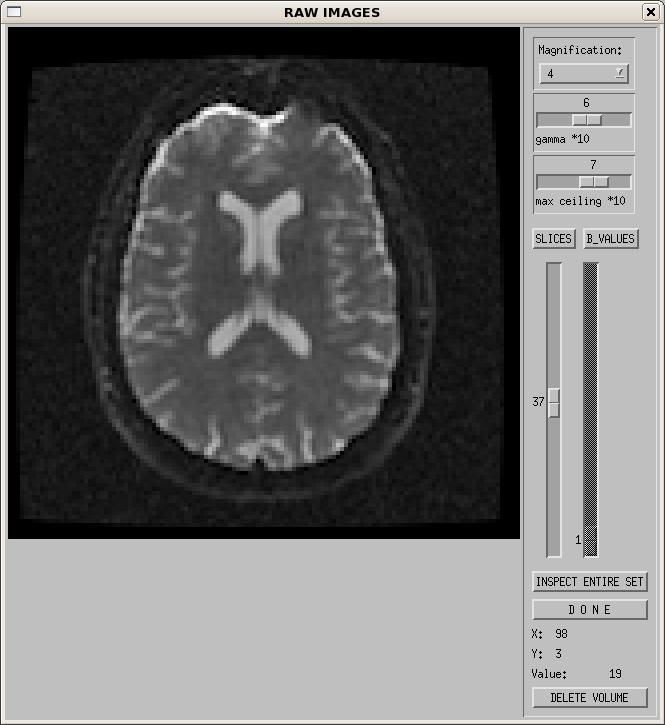
When you click on "Delete Volume,"" it removes whichever volume you are currently viewing (i.e. the b-value #). After you click that button, if you scan through the different b-values of your data, you should see 1 less volume than before.
When you click "Done" a new .list, .path and .bmtxt file are written out with the naming convention my_original_filename_DR.list, where _DR is added to indicate "data removed."
If you want to remove multiple volumes, be aware of the following items:
- After clicking "delete volume", the display refreshes. If you had a total of 42 volumes, and you removed volume #3, if you look now, there will still be a volume #3, but your top volume number is #41. In other words, all your volumes are re-numbered to be consecutive starting at volume #1. Thus, if you wish to remove multiple volumes, be aware that the volume numbers may have changed since you originally assessed your data.
- Tip: To avoid confusion with renumbering due to volume removal, start removing the volumes from the top (big numbered volumes) instead of the bottom (small numbered volumes). For example, if volume #3 and volume #40 are affected, remove volume #40 first such that the volume #39 is still #39.
- If you click "delete volume" then click "done" you will get a new listfile name _DR.list. If you then open the _DR.list file and decide to remove another volume by following the same procedure, you will get a new list file called _DR_DR.list. To avoid this, determine which volumes you wish to delete, and click on "delete volume" for each selected volume, then click "done". This will create a single new _DR.list file with only the final desired images.
- Note: If you remove a volume and click on the "inspect entire set" without clicking on the "done" button in the axial view, then the volume will not be removed and _DR.list file is not created. So it is important to click on the "done" button in the axial view after volume removal to record the detail.
- IMPORTANT: You will now need to run your DIFF_PREP processing on the _DR.list file! If you continue to use the my_original_filename.list then you will still be using data containing the un-wanted volumes.
- If you notice AFTER running DIFF_PREP that you want to remove volumes, load the _DMC.list file, and follow these rules:
- If I need to remove the first b0 image, I need to re-run DIFF_PREP because the registration makes use of the first b0 volume as a target for eddy and motion correction
- If I need to remove any volume OTHER than the first b0 volume, I need to run (or re-run) the tensor fitting in DIFF_CALC to create a tensor that does not include the un-wanted volumes. I DO NOT need to re-run DIFF_PREP pre-processing on this data.
- We want to re-iterate that if you find that you are removing large numbers of volumes, you may want to reconsider if it is worthwhile including this dataset in your analysis. Also, if you remove selected volumes from your data, please report this in all publications, so people are aware of the potential bias in FA values due to inconsistent numbers of images across your subjects1.
References
- Farrell JA, Landman BA, Jones CK, Smith SA, Prince JL, van Zijl PC, Mori S (2007) Effects of signal-to-noise ratio on the accuracy and reproducibility of diffusion tensor imaging-derived fractional anisotropy, mean diffusivity, and principal eigenvector measurements at 1.5 T. J Magn Reson Imaging. 26(3):756-67. abstract
- Tannazi, F., et al, (2011) Bias in Diffusion Tensor-Derived Quantities Depend on The Number of DWIs Composing The DT-MRI Dataset, 19th Annual ISMRM, Montreal, Quebec, Canada.